AI to the Aid of Humanitarian and Disaster Relief
Kathleen McKeown and Julia Hirschberg's automated system will help emergency teams break the code of little-studied languages in a hurry.
More than a quarter of the population of Nigeria uses smartphones, and a larger percentage has access to feature phones. In the case of a natural disaster or deadly disease outbreak, identifying areas for triage should in principle be easy, if only by monitoring social media posts. Yet because there are more than 500 languages in Nigeria, most of them uncategorized in terms of syntax, grammar, or lexicon, international disaster relief teams often run into an impenetrable barrier: the inability to understand these so-called low-resource languages (LRLs).
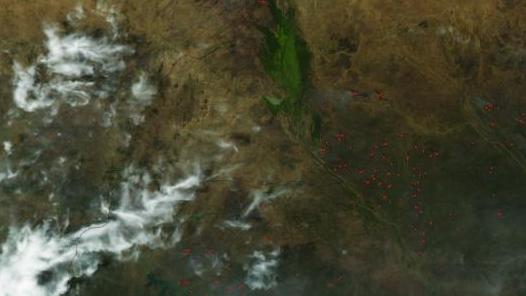
Kathleen McKeown and Julia Hirschberg’s language analysis work could help disaster response teams locate communities in distress. Satellite images can find potential risks, such as these hotspots in Nigeria that could be wildfires. McKeown and Hirschberg analyze the human reactions on the ground. (Image courtesy of NASA)
Millions of people around the globe speak a dazzling variety of such LRLs, making the task of disaster relief, from Africa to Asia, infinitely more complex. Working with a four-year Defense Advanced Research Projects Agency (DARPA) grant, Computer Science Professors Kathleen McKeown and Julia Hirschberg are leading the development of a universal sentiment and emotion detection system that will enable disaster relief workers confronted with an LRL to figure out who needs help the most, ideally within a day of their arrival in the region.
The Columbia project is part of a DARPA program called LORELEI, for low-resource languages for emergent incidents. While LORELEI technologies may include partial or fully automated speech recognition and/or machine translation, the overall goal will not be translating foreign language material into English but providing situation awareness by identifying elements of information in foreign language and English sources, such as topics, names, events, sentiments, and relationships. Situation awareness in such complex, dynamic scenarios implies an understanding and evaluation not just of the status of the disaster but of the causative events and the potential hazards ahead.
"The goal is to analyze the subjective posts and messages of people in crisis situations—whether they're feeling distress, urgency, anger—by accessing Twitter, the Web, news articles, and spoken language and to feed that information into our system, which would then identify the areas with the greatest need," explained McKeown, who is the Henry and Gertrude Rothschild Professor of Computer Science, director of the Data Science Institute at Columbia, and the principal investigator on the project.
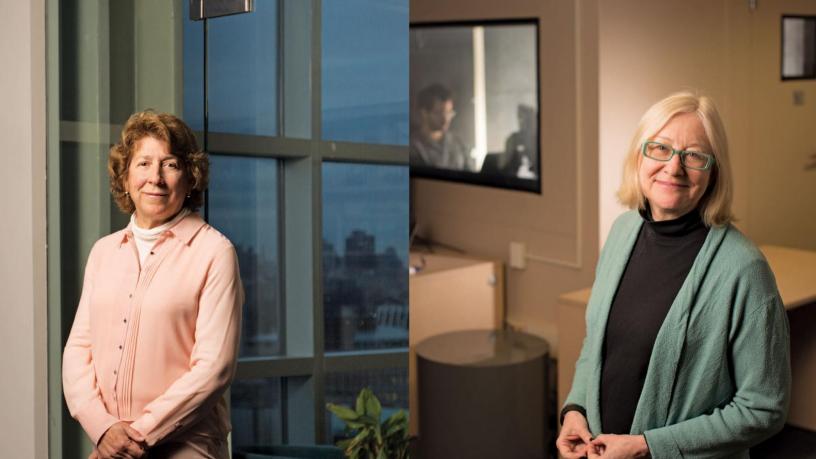
Kathy McKeown and Julia Hirschberg
Natural language processing systems learn through ingesting massive amounts of data. No data means no way to train the system, and the very term "low-resource language" basically states the team's major challenge. "Developing an automated system that generates a sentiment system for a new language is entirely new ground," McKeown said. "No one has really done it before."
The researchers, collaborating with colleagues from George Washington University, are attacking the problem from two directions: a supervised learning technique that employs novel methods of projection and a novel use of deep neural networks. The supervised learning approach uses speech data labeled for emotion in high-resource languages to train systems for identifying the same emotion in low-resource languages. With this technique, "we've shown that we can detect emotions such as anger and stress by training on one language and testing on another with performance about 17 percent above the baselines," said Hirschberg, who is the Percy K. and Vida L. W. Hudson Professor of Computer Science and chair of the Computer Science Department at Columbia.
In the second phase, the team is using a deep learning approach, training neural networks via cross-lingual word embeddings from both high-resource languages and a mix of low-resource languages. They then train a neural net using the cross-lingual embeddings and data that are labeled in English. The researchers also have developed techniques that enable automatic generation of a lexicon that tags words or phrases in a new language, such as the Uyghur language spoken in rural China, with the emotions they express. McKeown and Hirschberg will be working with their team to produce systems that can recognize sentiment and emotion in a variety of underresourced languages.